Issue #1: Introduction, PAC Isometry, Over-Smoothing, and Evolution of the Field.

Welcome!
Three years ago Sebastian Ruder started his NLP newsletter with the words that “NLP is seeing increasing interest recently”. Today NLP is at the peak 🗻 of its popularity, featured across all major media sources.
I hope that Graph Machine Learning (which I abbreviate as GML) is in the same state as NLP was three years ago. Indeed, the number of GML papers posted on ArXiv CS section is more 300 each month (!) and all major conferences have a decent portion of papers in the intersection of graphs and machine learning.
The goal for this newsletter is quite simple: I want people to know more about recent breakthroughs, current trends, and future events in GML. I already post daily in telegram about GML and this newsletter can be considered as a less frequent, more condensed version of what I run there. Besides, I make blog posts on Medium and tweet on twitter (feel free to follow).
This email is about how can we design theoretically better GNN models and what stands in the way of deep GNN. You can also find digests of recent conferences that were rich on graph-related topics and many releases and updates of GML libraries that will make your life easier🧙♂️.
Let’s go!
Blog posts
In a series of blog posts, Michael Bronstein touches several important points regarding the developments of new Graph Neural Networks (GNN). One is the fact that most of the current approaches to study the expressive power of GNN was on the comparison of GNN against its algorithmic counterpart, Weisfeiler-Lehman (WL) algorithm. However, this is not necessarily the most useful comparison as it ignores the case when the two graphs are similar but not isomorphic.
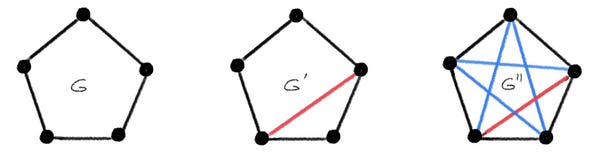
Instead of asking GNN to distinguish graphs by isomorphism testing, a more general way to compare expressive power of GNNs is by probably approximate isometry: 𝖯( c⁻¹ d(G,G′)−ε ≤ |f(G)−f(G′)| ≤ c d(G,G′) )>1−δ
Another question that he raises is why deep GNNs are not the thing for GML to the same extent as deep CNNs are for CV. There is a profound reason for that, termed over-smoothing, that makes node embeddings indistinguishable from each other with the growth of the number of layers. There are ways to overcome it by different means such as regularization; yet, it still does not show any significant improvement in real-world settings. As outlined, we may just miss proper data sets where the target labels strongly depend on higher-order graph structures.

While there are ways to "fix" performance of typical GNNs with more layers, the overall deep GNN models do not bring significant advantage yet.
You can find more interesting posts by Michael Bronstein on this page.
Finally, if you are a little bit rusty or just started studying graphs check out this quick and intuitive post on centrality measures by Anas Ait Aomar.
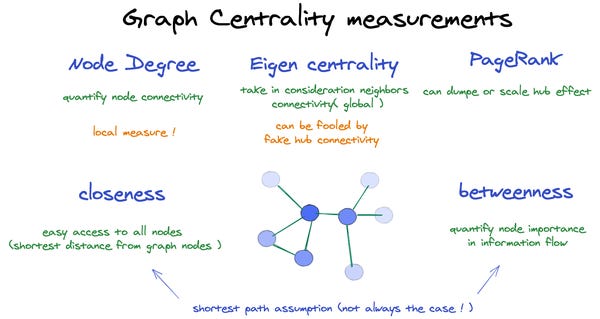
Podcasts
In a recent TWIML podcast 🎧 Michael Bronstein compared his views on the field 2 years ago vs today: fast evolution with the appearance of software/hardware/datasets; the difference between academic and industrial research; his recent papers as well as his vision of the field for the next 5 years.
Conferences
Several big events recently took place that presented the latest advancements of the field from different perspectives.
One of the top 🔝 conferences ICML has a variety of GML papers ranging from structural design 👷♂️ to linear solvers to particle dynamics. Besides the main track at ICML there were two relevant graph workshops, GRL+ (more on it below) and Bridge Between Perception and Reasoning (videos are here), each featuring novel perspectives on how graphs capture different aspects of reality.
Graph Representation Learning and Beyond (GRL➕) workshop at ICML is a venue for researchers to discuss cutting-edge ideas and perspectives of GML. Current trends in GML include topics such as emerging work on performance/scalability, stronger KG embeddings, a proposal of many datasets/benchmarks/libraries, and applications to computational chemistry and algorithmic reasoning. Besides, Petar Veličković (one of the organizers) mentions explicit consideration of structure for GNN as the main standout, not only in the papers but also in most of the invited talks. Videos are here.
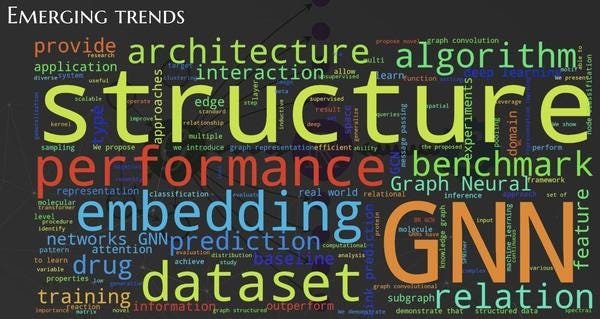
Another top conference ACL in NLP ✍ also had a solid record of papers with a graph in its title (in total around 50 papers (8% of total)). Knowledge graphs play a big role in several applications such as chatbots and as Michael Galkin put it in a blog post the main theme of this year was discovering that knowledge graphs demonstrate better capabilities to reveal higher-order interdependencies in otherwise unstructured data.
Automated Knowledge Base Construction (AKBC) conference had its second edition (previously held as part of bigger conferences). In total 29 papers present research on extracting entities and relationships in various sources of data (text, images, video, etc.) and then using it to deliver insights to the users 🙌. You can find the videos on YouTube as well as highlights of AKBC 2020 from Daniel Daza, which features papers on embedding complex queries, inductive representation learning in KGs, and KGs for information extraction.
In August there will be another big conference KDD with a line-up of great papers and 4 (!) workshops (1, 2, 3, 4) on GML. I encourage someone to spend some time digesting and writing a blog post about GML works at KDD (which I will feature in the next email) 🙏.
Software
There are some major updates on software libraries 👩💻. PyTorch-Geometric released version 1.6.0 with several improvements for scalability such as support for mini-batch training, memory-efficient graph storage SparseTensor, and conversion to TorchScript programs. For more check out this video by Matthias Fey.
Another popular library DGL aims to provide software that offers flexibility for different real-world domains such as knowledge graphs, chemistry and biology, CV and NLP, as well as prod-ready distributed training. More details can be found in this video by Zheng Zhang.
Besides these big 2️⃣, there were numerous new libraries that serve specific needs of using graph models. Geo2DR is GPU-ready library for unsupervised learning on graph embeddings through substructure decomposition. Spektral is a library for graph deep learning, based on the Keras API and TensorFlow 2. Graphein is a library for constructing graph and surface-mesh representations of protein structures for computational analysis. Geoopt is a PyTorch add-on that allows you to perform Riemannian optimization for neural network models. PyKEEN is a python package designed to train and evaluate knowledge graph embedding models. Additionally, several graph data sets have been updated and formalized: Wiki-CS, OGB, TUDataset.
That’s it folks for today.
Feedback 💬 If you have some opinion how to improve this issue, feel free to reply to this email. Likewise, contribute 💪to the future newsletter if you have content that can be relevant to the community. And please spread the word among your friends by emailing this letter or by tweeting about it 🐦